Personalakten werden häufig noch in Papier geführt
Zu jedem Arbeitsverhältnis in Deutschland gehört eine Personalakte. Viele liegen nach wie vor in Papier vor. Während die einen noch aktiv bearbeitet werden, dienen Altakten der Nachweispflicht und nehmen im Wesentlichen nur noch Platz weg. Digitale Akten haben zahlreiche Vorteile: Höherer Bearbeitungskomfort, weniger Raumbedarf oder leichtere Zugriffssteuerung. Eine besondere Bedeutung kommt dem Datenschutz zu. Personenbezogenen Daten müssen nach bestimmten Fristen gelöscht werden. Auch wenn es keine direkten Aufbewahrungsfristen für Personalakten gibt, ergeben sich diese mittelbar aus Gesetzten wie dem BGB, dem Einkommensteuergesetz oder dem Sozialgesetzbuch. Diese Fristen liegen zwischen 3 und 30 Jahren und betreffen jeweils einzelne Dokumente der Personalakte, nicht die gesamte Akte. Daher muss streng genommen jede Papierakte regelmäßig bewertet werden, ob Teile vernichtet werden müssen. Andernfalls drohen Strafen für unrechtmäßige Datenspeicherung. Bei digitalen Dokumenten ist dieser Prozess deutlich einfacher.
Warum Digitalisierung von Personalakten sinnvoll ist
Vieles spricht also für eine Digitalisierung der papiergebundenen Personalakten. Es gibt am Markt viele Dienstleister, die Akten einscannen und als elektronische Dokumente zur Verfügung stellen. Damit können die einzelnen Dokumente in das DMS (Dokumentenmanagementsystem) importiert und den entsprechenden Personen und Bearbeitern zugeordnet werden. Um die oben erwähnten Löschfristen sicherzustellen und den Bearbeitungskomfort zu erhöhen, müssen allerdings die Einzeldokumente hinreichend klassifiziert werden.
Die eigentliche Digitalisierung der Akten ist keine besonders schwierige Aufgabe. Moderne Scanner schaffen ein paar hundert Blatt pro Minute.
Manuelle Vorverarbeitung ist aufwändig und teuer
Der Hauptaufwand entsteht durch die notwendige manuelle Vorverarbeitung der zu digitalisierenden Akten. Üblicherweise übernehmen regelbasierte Systeme diese Aufgabe nach der Digitalisierung anhand der Bilddateien. Dazu programmiert ein Mensch, wie Dokumente erkannt werden sollen. Für eine hohe Automatisierungsrate mit niedrigen Kosten sind homogene Dokumentenstrukturen und eine einheitliche Qualität ebenso notwendig wie wiederverwendbare Dokumentenklassen der Unternehmen.
Bei Personalakten bestehen in der Regel spezielle Herausforderungen, die eine Automatisierung behindern:
- Die Akten sind über Jahre gewachsen und daher nicht immer in bester Qualität, wodurch die Lesbarkeit beeinträchtigt ist.
- Handschriftliche Anmerkungen gehören zu fast jeder Personalakte und können de facto nicht maschinell verarbeitet werden.
- Die Dokumente verändern sich hinsichtlich Layout und Struktur im Laufe der Zeit, so dass viele Varianten von eigentlich identischen Dokumentenklassen vorliegen.
- Die Ablageordnung unterscheidet sich von Unternehmen zu Unternehmen. Daher kann kaum ein Standardschema zur Trennung programmiert werden, sondern jedes Projekt fängt bei Null an.
- Die Unternehmen geben Dokumentenklassen häufig vor und richten sich gerne nach dem Prinzip „Wünschdirwas“. Keine Chance auf Wiederverwendung der Schemata zwischen Projekten.
Typischer Prozess der Aktendigitalisierung mit manueller Scanvorbereitung
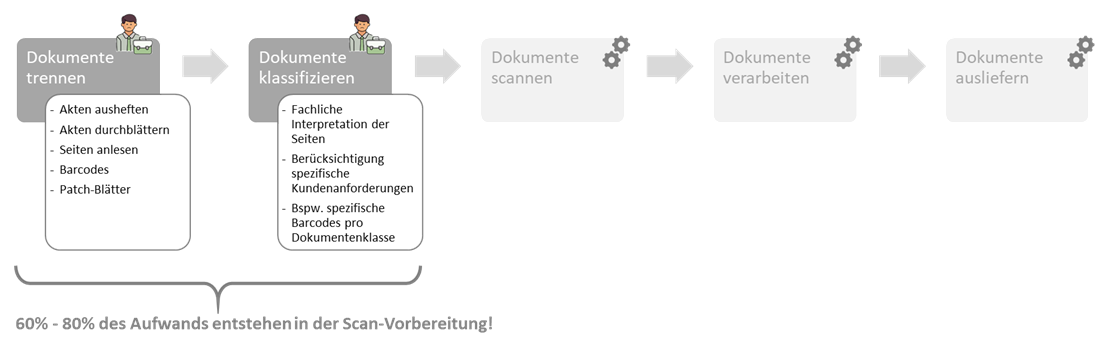
Trennung und Klassifikation sind aus den oben genannten Gründen nur schwer zu automatisieren. In der Folge wird oft auf einen manuellen Prozess zurückgegriffen: Mitarbeiter trennen manuell vor dem Scannen die Dokumente mit Trennblättern oder Barcodes. Die Klassifikation erfolgt in dem Prozess ebenfalls manuell.
Im Ergebnis ist das Digitalisieren von Personalakten vergleichsweise teuer – für einige interessierte Kunden zu teuer, da nicht wirtschaftlich. Durch die fachliche Qualifikation, die zum Bearbeiten von Personaldokumenten notwendig ist, stehen außerdem teilweise nicht genug Kapazitäten zur Verfügung. Lange Wartezeiten sind üblich oder Kundenanfragen werden nicht bedient.
Erfahren Sie mehr über Ihre Vorteile von Automatisierung mit KI in unserem Web-Seminar
Bei Dokumenten mit geringerer Struktur und komplexen fachlichen Anforderungen bieten Cloud-basierte Plattformen mit Machine Learning und KI die Chance, gute Extraktionsergebnisse in kurzer Zeit und zu geringen Stückkosten zu erstellen. Wie das geht erfahren Sie im Web-Seminar!
Lassen Sie Maschinen den Prozess lernen!
Künstliche Intelligenz und Machine Learning können die gesamte Verarbeitung eingehender Dokumente in kurzer Zeit mit wenigen Beispielen lernen und auf neue Dokumente anwenden. Ausgangspunkt ist ein Paradigmenwechsel im Vergleich zur bisherigen Arbeitsweise:
- Spezialisierung: Statt umfassende Anwendungen zu programmieren, die den gesamten Prozess abdecken, werden KI-Services als Bausteine jeweils für spezielle Aufgaben eingesetzt. Diese lassen sich schneller und besser trainieren, als umfassende Funktionen, die „alles“ können sollen.
- Trainieren statt Programmieren: Statt Regeln zu programmieren, lernen intelligente Systeme die Verarbeitungsschritte von einem fachlichen Experten oder Trainer anhand weniger Beispiele. So lässt sich schnelle Umsetzung mit hoher Flexibilität kombinieren.
- Human in the loop: Anhand ihrer Sicherheit (Konfidenz) schlägt die KI im Training Beispiele vor und sondert in der Dunkelverarbeitung Fälle aus. So entsteht ein kontinuierlicher Verbesserungsprozess mit minimierten Aufwand und der Mensch behält die Kontrolle über den Prozess und dessen Qualität.
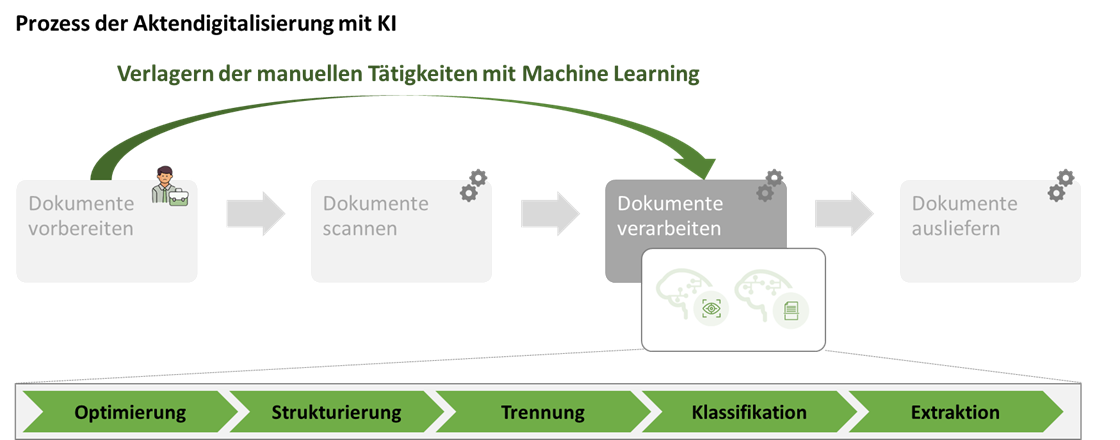
Mit KI mehr erreichen mit weniger Aufwand
Moderne KI-Plattformen wenden die genannten Grundsätze an und kombinieren einzelne, spezielle KI-Services zu einem effizienten und effektiven Gesamtprozess. Bezogen auf den Digitalisierungsprozess von Personalakten kann mit Machine Learning ein Großteil der manuellen Arbeit aus der Scan-Vorbereitung in die digitale Nachbereitung verlagert und automatisiert ausgeführt werden:
- Optimierung: KI lernt, Nutzdaten von Störungen auf Dokumenten zu unterscheiden. Optische Störungen, wie bspw. vergilbte Papierfarben oder Urkundenhintergründe, können kompensiert werden, um Texterkennung und Extraktion zu verbessern.
- Strukturierung: KI-Bausteine gruppieren ohne menschliches Zutun: Die Dokumente werden bereits nach visuellen und textlichen Merkmalen gruppiert, um den weiteren Verarbeitungsprozess zielgerichtet zu steuern. Damit werden bestimmte wiederkehrende Dokumententypen mit einer hohen Automatisierungsquote in sogenannte Cluster zusammengefasst.
- Trennung: Anhand von Mustern erlernen KI-Systeme eingehende Dokumente automatisch zu trennen. Dazu verwendet die KI selbstständig Seitennummern, Layoutinformationen und optische Merkmale.
- Klassifikation: Ebenfalls anhand von Beispielen erlernen KI-Systeme Dokumentenklassen mit hoher Genauigkeit zu erkennen. Und das unabhängig von Layout oder Schlagworten.
- Extraktion: Indem auf einigen Trainingsdokumenten relevante Daten durch einen Trainer markiert werden, lernt die KI, relevante Fachdaten auf allen weiteren Dokumenten automatisch zu erkennen.
Durch eine geschickte Kombination der oben beschriebenen Services lassen sich sehr kurze Umsetzungs- und Anlernphasen mit niedrigen Stückkosten kombinieren.
Fazit: Schnellere Digitalisierung von Personalakten mit KI bei gleichzeitig niedrigeren Kosten
Durch den kombinierten Einsatz mehrerer KI- bzw. Machine Learning-Methoden kann mit weniger Aufwand mehr Qualität bei der Digitalisierung von Personalakten erreicht werden:
- Durch die Automatisierung der Bearbeitungsschritte wird die Scan-Vorbereitung entlastet und die Kosten deutlich gesenkt.
- Durch die bessere Erkennungsqualität der KI sinken Aufwand und Kosten für die manuelle Nachbereitung zusätzlich.
- Durch sinkende Kosten können niedrigere Preise realisiert und zusätzliche Kunden gewonnen werden, für die eine Digitalisierung der Personalakten bisher nicht wirtschaftlich war.
- Durch den geringeren Personalbedarf an Fachkräften können Kapazitätsengpässe beseitigt werden.
- Durch die zusätzlichen Kapazitäten können auch sehr große Aufträge schneller abgearbeitet werden.
Die Einführung und Nutzung solcher Lösungen erfordert kein mehrjähriges Projekt und nur vergleichsweise geringe Investitionen. Mit leistungsfähigen, service-orientierten Architekturen wie der inserve-Plattform lassen sich Digitalisierungsprozesse für Personal und andere Akten in kurzer Zeit automatisieren.
Fragen oder Anmerkungen? Wir freuen uns auf Ihr Feedback zum Artikel und einen persönlichen Austausch.
Direkt Termin vereinbaren!
Mit dem Online-Tool Calendly können Sie direkt online Ihren Wunschtermin wählen. Buchen Sie noch heute Ihre 15-minütige Besprechung bei Dietmar Neidig und erhalten Sie eine Kalender-Einladung mit Zugangslink zu Ihrer persönlichen Teams-Besprechung.
